

13 Greater density of BMI-healthy stores was found to be significantly associated with reduced BMI. 15 Also in the USA, food stores were classified as either BMI-healthy, BMI-intermediate, or BMI-unhealthy, and kernel density estimates of the distribution of each of these was examined in relation to BMI. 15 These findings led them to conclude that the food environment can have differential effects on residents depending on the pressures placed on individual financial resources. 15 They found that for higher income groups, a healthier food environment was associated with lower rates of obesity, but for lower income groups, higher rates of obesity were observed among those living in a healthier food environment. 13, 15 Among a sample of adults with diabetes, one study created a food environment score by subtracting the kernel density estimate of unhealthy food stores from that of healthy food stores, and examined associations between the food environment score and obesity. In the USA, researchers have applied KDE methods to the examination of the relationship between the intensity/density of elements of the built environment and BMI, or obesity.
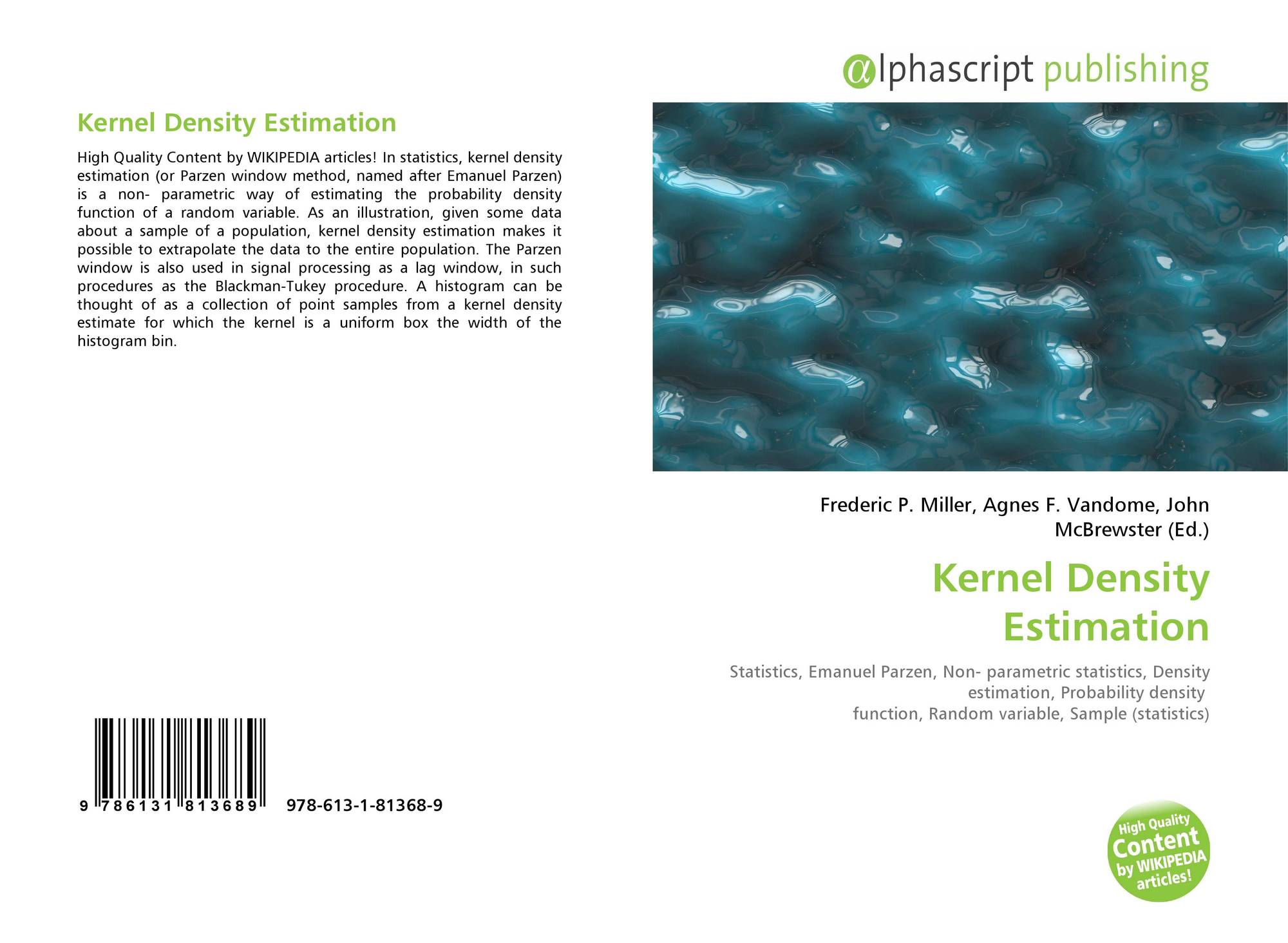
There is a paucity of studies that have used KDE to examine the relationship between destinations and BMI. 14 In plain terms, the use of KDE to examine the distribution of destinations in neighbourhoods enables researchers to see where destinations are sparsely distributed (dispersed), and where they are more intensely distributed (clustered). Further, by representing the distribution of activity or exposures on a continuous surface, KDE helps identify the presence of clusters and irregularities. 12, 13 The ability to weight the distribution of destinations according to their proximity to a central feature or location is one of the key imperatives for the use of KDE. Kernel density estimation (KDE)-a spatial analysis technique that accounts for the location of features (ie, destinations) relative to each other-is an emerging spatial tool that has previously been applied to the examination of various aspects of the environment, such as park access, 10 health resources, 11 and recently, the food environment. Furthermore, such measures of destination accessibility do not take into account the location of destinations relative to each other (ie, they provide no indication of whether they are intensely distributed or dispersed). 9, 10 A destination located at the edge of the areal unit is not equivalent to a more proximal destination, however, typical binary measures do not accommodate this, and analyse them as if their effect is the same. Such measures have been criticised on the basis of their binary or categorical classification: a feature (in this case destination) is simply classified as present or absent. Most commonly, access to destinations in neighbourhoods has been measured in terms of the destinations present within a defined catchment or buffer (ie, a count of the number of destinations within a certain distance of home, or the presence of destinations within a defined area). The limitations of standard approaches in operationalising elements of the built environment may explain some of the contradictory findings. 3 Examination of destinations, an increasingly common focus of neighbourhood research, has yielded mixed results: inverse relationships between body mass index (BMI) and grocery or supermarket store availability have been observed in some research, 4–6 while positive relationships have been noted elsewhere between BMI and destinations such as small food stores and supermarkets, 7 and fast-food stores. While it seems plausible that the rise in obesity can be partly attributed to the built environment, the abundant literature examining aspects of the built environment in relation to weight status has yielded equivocal results, with calls for better metrics to evaluate associations. 2 There is growing interest in understanding how the neighbourhood environment may influence the risk of overweight and obesity by encouraging increased energy consumption and discouraging energy expenditure. 1 Among developed countries, the economic costs associated with overweight and obesity are significant. Obesity remains a growing problem in many Western countries including Australia, where 63% of the adult population is overweight or obese.
